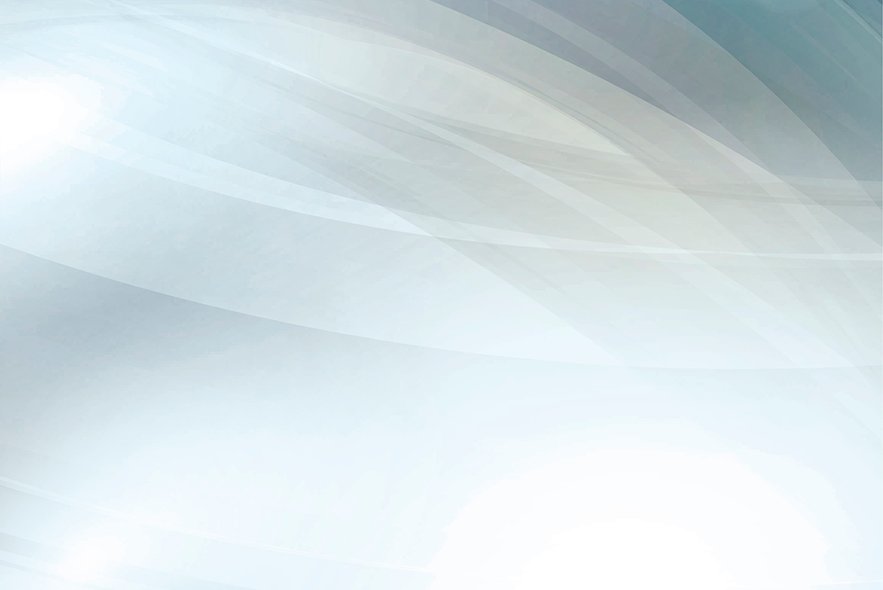
deep learning
What is deep learning?
Deep learning is a type of machine learning and artificial intelligence (AI) that imitates the way humans gain certain types of knowledge. Deep learning models can be taught to perform classification tasks and recognize patterns in photos, text, audio and other various data. It is also used to automate tasks that would normally need human intelligence, such as describing images or transcribing audio files.
Deep learning is an important element of data science, including statistics and predictive modeling. It is extremely beneficial to data scientists who are tasked with collecting, analyzing and interpreting large amounts of data; deep learning makes this process faster and easier.
Where human brains have millions of interconnected neurons that work together to learn information, deep learning features neural networks constructed from multiple layers of software nodes that work together. Deep learning models are trained using a large set of labeled data and neural network architectures.
Deep learning enables a computer to learn by example. To understand deep learning, imagine a toddler whose first word is dog. The toddler learns what a dog is -- and is not -- by pointing to objects and saying the word dog. The parent says, "Yes, that is a dog," or, "No, that is not a dog." As the toddler continues to point to objects, he becomes more aware of the features that all dogs possess. What the toddler is doing, without knowing it, is clarifying a complex abstraction: the concept of dog. They are doing this by building a hierarchy in which each level of abstraction is created with knowledge that was gained from the preceding layer of the hierarchy.
Why is deep learning important?
Deep learning requires both a large amount of labeled data and computing power. If an organization can accommodate for both needs, deep learning can be used in areas such as digital assistants, fraud detection and facial recognition. Deep learning also has a high recognition accuracy, which is crucial for other potential applications where safety is a major factor, such as in autonomous cars or medical devices.
How deep learning works
Computer programs that use deep learning go through much the same process as a toddler learning to identify a dog, for example.
Deep learning programs have multiple layers of interconnected nodes, with each layer building upon the last to refine and optimize predictions and classifications. Deep learning performs nonlinear transformations to its input and uses what it learns to create a statistical model as output. Iterations continue until the output has reached an acceptable level of accuracy. The number of processing layers through which data must pass is what inspired the label deep.
In traditional machine learning, the learning process is supervised, and the programmer must be extremely specific when telling the computer what types of things it should be looking for to decide if an image contains a dog or does not contain a dog. This is a laborious process called feature extraction, and the computer's success rate depends entirely upon the programmer's ability to accurately define a feature set for dog. The advantage of deep learning is the program builds the feature set by itself without supervision.
Initially, the computer program might be provided with training data -- a set of images for which a human has labeled each image dog or not dog with metatags. The program uses the information it receives from the training data to create a feature set for dog and build a predictive model. In this case, the model the computer first creates might predict that anything in an image that has four legs and a tail should be labeled dog. Of course, the program is not aware of the labels four legs or tail. It simply looks for patterns of pixels in the digital data. With each iteration, the predictive model becomes more complex and more accurate.
Unlike the toddler, who takes weeks or even months to understand the concept of dog, a computer program that uses deep learning algorithms can be shown a training set and sort through millions of images, accurately identifying which images have dogs in them, within a few minutes.
To achieve an acceptable level of accuracy, deep learning programs require access to immense amounts of training data and processing power, neither of which were easily available to programmers until the era of big data and cloud computing. Because deep learning programming can create complex statistical models directly from its own iterative output, it is able to create accurate predictive models from large quantities of unlabeled, unstructured data.
Deep learning methods
Various methods can be used to create strong deep learning models. These techniques include learning rate decay, transfer learning, training from scratch and dropout.
Learning rate decay
The learning rate is a hyperparameter -- a factor that defines the system or sets conditions for its operation prior to the learning process -- that controls how much change the model experiences in response to the estimated error every time the model weights are altered. Learning rates that are too high may result in unstable training processes or the learning of a suboptimal set of weights. Learning rates that are too small may produce a lengthy training process that has the potential to get stuck.
The learning rate decay method -- also called learning rate annealing or adaptive learning rate -- is the process of adapting the learning rate to increase performance and reduce training time. The easiest and most common adaptations of learning rate during training include techniques to reduce the learning rate over time.
Transfer learning
This process involves perfecting a previously trained model; it requires an interface to the internals of a preexisting network. First, users feed the existing network new data containing previously unknown classifications. Once adjustments are made to the network, new tasks can be performed with more specific categorizing abilities. This method has the advantage of requiring much less data than others, thus reducing computation time to minutes or hours.
Training from scratch
This method requires a developer to collect a large, labeled data set and configure a network architecture that can learn the features and model. This technique is especially useful for new applications, as well as applications with many output categories. However, overall, it is a less common approach, as it requires inordinate amounts of data, causing training to take days or weeks.
Dropout
This method attempts to solve the problem of overfitting in networks with large amounts of parameters by randomly dropping units and their connections from the neural network during training. It has been proven that the dropout method can improve the performance of neural networks on supervised learning tasks in areas such as speech recognition, document classification and computational biology.
Deep learning neural networks
A type of advanced machine learning algorithm, known as an artificial neural network (ANN), underpins most deep learning models. As a result, deep learning may sometimes be referred to as deep neural learning or deep neural network (DDN).
DDNs consist of input, hidden and output layers. Input nodes act as a layer to place input data. The number of output layers and nodes required change per output. For example, yes or no outputs only need two nodes, while outputs with more data require more nodes. The hidden layers are multiple layers that process and pass data to other layers in the neural network.
Neural networks come in several different forms, including the following:
- Recurrent neural networks.
- Convolutional neural networks.
- ANNs and feed.
- Forward neural networks.
Each type of neural network has benefits for specific use cases. However, they all function in somewhat similar ways -- by feeding data in and letting the model figure out for itself whether it has made the right interpretation or decision about a given data element.
Neural networks involve a trial-and-error process, so they need massive amounts of data on which to train. It's no coincidence neural networks became popular only after most enterprises embraced big data analytics and accumulated large stores of data. Because the model's first few iterations involve somewhat educated guesses on the contents of an image or parts of speech, the data used during the training stage must be labeled so the model can see if its guess was accurate. This means unstructured data is less helpful. Unstructured data can only be analyzed by a deep learning model once it has been trained and reaches an acceptable level of accuracy, but deep learning models can't train on unstructured data.
Deep learning benefits
Benefits of deep learning include the following:
- Automatic feature learning. Deep learning systems can perform feature extraction automatically, meaning they don't require supervision to add new features.
- Pattern discovery. Deep learning systems can analyze large amounts of data and uncover complex patterns in images, text and audio and can derive insights that it might not have been trained on.
- Processing of volatile data sets. Deep learning systems can categorize and sort data sets that have large variations in them, such as in transaction and fraud systems.
- Data types. Deep learning systems can process both structured and unstructured data.
- Accuracy. Any additional node layers used aid in optimizing deep learning models for accuracy.
- Can do more than other machine learning methods. When compared to typical machine learning processes, deep learning needs less human intervention and can analyze data that other machine learning processes can't do as well.
Deep learning examples
Because deep learning models process information in ways similar to the human brain, they can be applied to many tasks people do. Deep learning is currently used in most common image recognition tools, natural language processing (NLP) and speech recognition software.
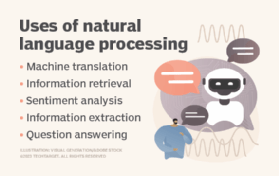
Use cases today for deep learning include all types of big data analytics applications, especially those focused on NLP, language translation, medical diagnosis, stock market trading signals, network security and image recognition.
Specific fields in which deep learning is currently being used include the following:
- Customer experience (CX). Deep learning models are already being used for chatbots. And, as it continues to mature, deep learning is expected to be implemented in various businesses to improve CX and increase customer satisfaction.
- Text generation. Machines are being taught the grammar and style of a piece of text and are then using this model to automatically create a completely new text matching the proper spelling, grammar and style of the original text.
- Aerospace and military. Deep learning is being used to detect objects from satellites that identify areas of interest, as well as safe or unsafe zones for troops.
- Industrial automation. Deep learning is improving worker safety in environments like factories and warehouses by providing services through industrial automation that automatically detect when a worker or object is getting too close to a machine.
- Adding color. Color can be added to black-and-white photos and videos using deep learning models. In the past, this was an extremely time-consuming, manual process.
- Computer vision. Deep learning has greatly enhanced computer vision, providing computers with extreme accuracy for object detection and image classification, restoration and segmentation.
Limitations and challenges
Deep learning systems come with downsides as well, for example:
- They learn through observations, which means they only know what was in the data on which they trained. If a user has a small amount of data or it comes from one specific source that is not necessarily representative of the broader functional area, the models do not learn in a way that is generalizable.
- The issue of biases is also a major problem for deep learning models. If a model trains on data that contains biases, the model reproduces those biases in its predictions. This has been a vexing problem for deep learning programmers as models learn to differentiate based on subtle variations in data elements. Often, the factors it determines are important are not made explicitly clear to the programmer. This means, for example, a facial recognition model might make determinations about people's characteristics based on things like race or gender without the programmer being aware.
- The learning rate also becomes a major challenge to deep learning models. If the rate is too high, then the model converges too quickly, producing a less-than-optimal solution. If the rate is too low, then the process may get stuck, and it is even harder to reach a solution.
- The hardware requirements for deep learning models also create limitations. Multicore high-performing graphics processing units (GPUs) and other similar processing units are required to ensure improved efficiency and decreased time consumption. However, these units are expensive and use large amounts of energy. Other hardware requirements include RAM and a hard disk drive or RAM-based solid-state drive.
Other limitations and challenges include the following:
- Requires large amounts of data. Furthermore, the more powerful and accurate models need more parameters, which, in turn, require more data.
- Lack of multitasking. Once trained, deep learning models become inflexible and cannot handle multitasking. They can deliver efficient and accurate solutions but only to one specific problem. Even solving a similar problem would require retraining the system.
- Lack of reasoning. Any application that requires reasoning -- such as programming or applying the scientific method -- long-term planning and algorithm-like data manipulation is completely beyond what current deep learning techniques can do, even with large amounts of data.
Deep learning vs. machine learning
Deep learning is a subset of machine learning that differentiates itself through the way it solves problems. Machine learning requires a domain expert to identify most applied features. On the other hand, deep learning understands features incrementally, thus eliminating the need for domain expertise.
This makes deep learning algorithms take much longer to train than machine learning algorithms, which only need a few seconds to a few hours. However, the reverse is true during testing. Deep learning algorithms take much less time to run tests than machine learning algorithms, whose test time increases along with the size of the data.
Furthermore, machine learning does not require the same costly, high-end machines and high-performing GPUs that deep learning does.
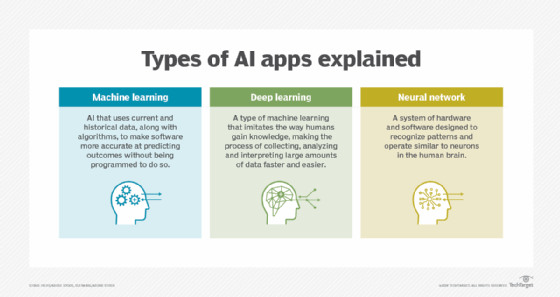
In the end, many data scientists choose traditional machine learning over deep learning due to its superior interpretability, or the ability to make sense of the solutions. Machine learning algorithms are also preferred when the data is small.
Instances where deep learning becomes preferable include situations where there is a large amount of data, a lack of domain understanding for feature introspection or complex problems, such as speech recognition and NLP.
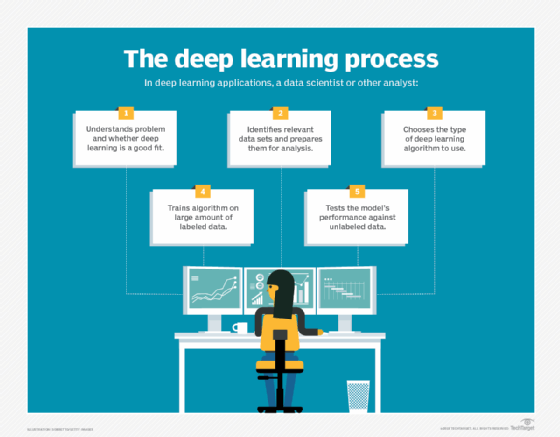
Potential applications of deep learning in the future
Currently, deep learning is used in common technologies, such as in automatic facial recognition systems, digital assistants and fraud detection. Deep learning is also used in emerging technologies as well.
For example, it is used in the medical field to detect delirium in critically ill patients. Cancer researchers have also started implementing deep learning into their practice as a way to automatically detect cancer cells. Self-driving cars are also using deep learning to automatically detect objects such as road signs or pedestrians. And social media platforms can use deep learning for content moderation, combing through images and audio.
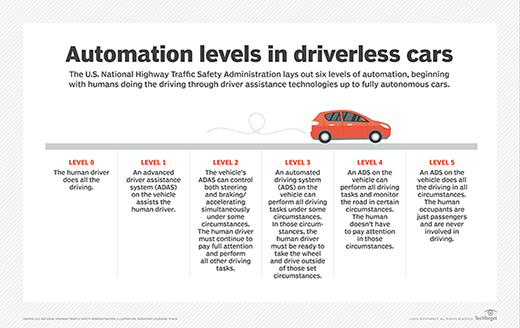
Learn more about how deep learning compares to machine learning and other forms of AI.